Arjun Karuvally
theory. intelligence. computation. math. philosophy.
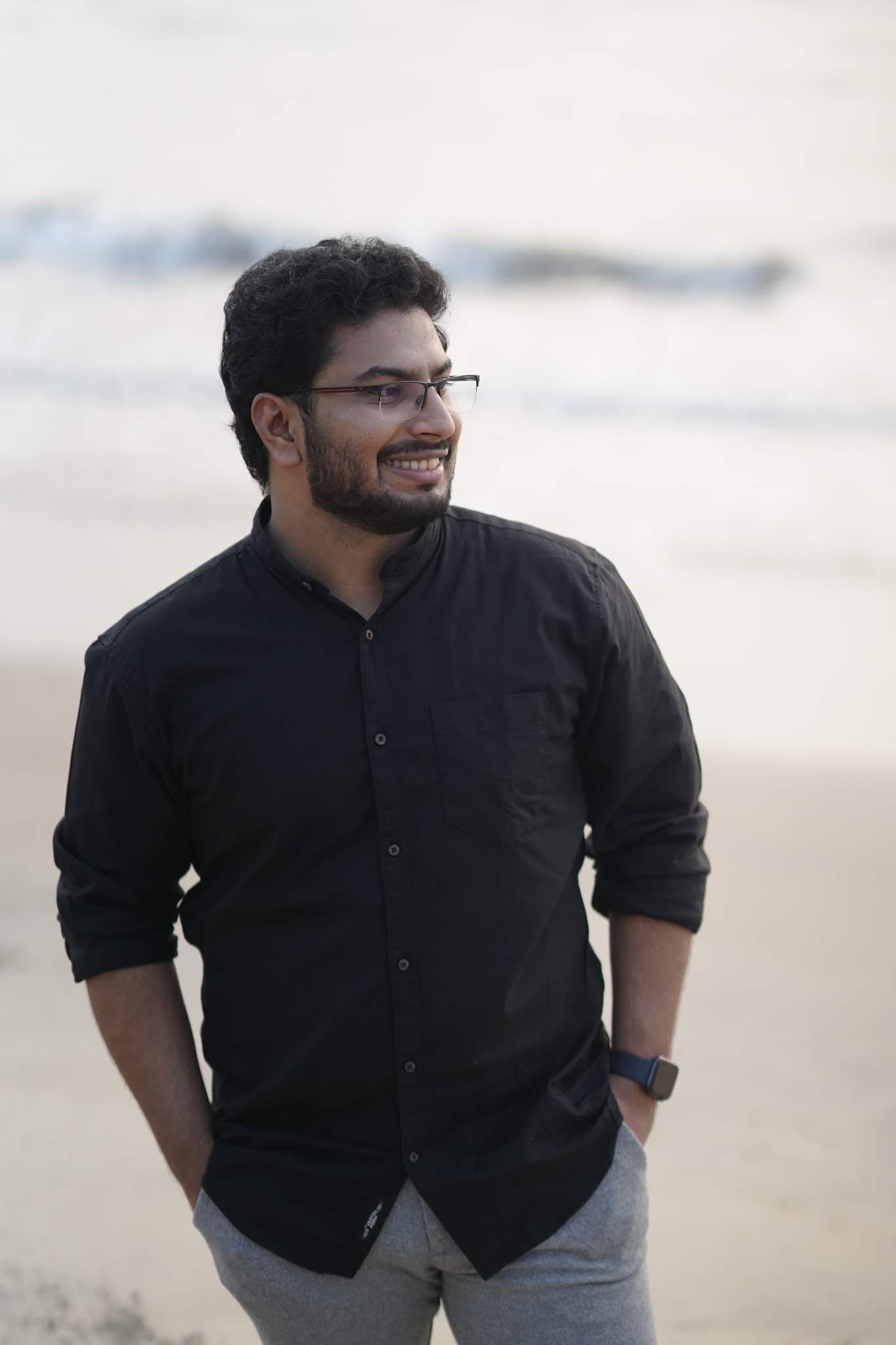
PhD. Candidate
BiNDS Laboratory, UMASS
Hello and welcome! I’m a scientist with a curiosity for unraveling the mysteries that link the human mind and artificial intelligence. But let’s not get ahead of ourselves; allow me to walk you through my journey—a tale of math, code, and a quest for understanding that goes beyond mere equations.
The Allure of Many Fields Since the start of my academic journey, I have been enamored by a multitude of disciplines—ranging from the intricacies of human intelligence to the abstract beauty of mathematical models and the thought-provoking landscapes of scientific philosophy.
Memory Models After diving headfirst into artificial intelligence, I found my interest in memory models. In my opinion, memory can potentially decode how intelligence—both artificial and human—functions. I believe that memory isn’t just a storehouse of information; it’s the foundational bedrock upon which intelligence is built. Understanding memory in its most fundamental manner unlocks the secrets of cognition and intelligent behavior.
Current Focus: Beyond The Black Box Now, if you’ve ever delved into artificial neural networks, you’ve likely heard them referred to as ‘black boxes,’ mysterious entities that perform complex calculations yet resist easy explanation. My current research aims to shed light into them. I focus on what is called mechanistic interpretability, which is a fancy way of saying I want to know the “how” and the “why” behind these computational models, not just the “what.”
I am trying to uncover universal computations—common threads in various models that may be integral to their functioning. Think of it this way: A car mechanic doesn’t merely know how to change the oil; they understand the entire engine, inside and out. That’s the level of comprehension I aim for with neural networks. If we’re going to trust these systems with increasingly complex tasks, we should understand their inner workings to the same degree that a mechanic understands a car engine.
The Horizon: A Mechanic for The Mind? As we gaze into the future, I envision a world where artificial neural networks and human cognition are not mystical phenomena, but well-understood systems. Systems that can be diagnosed, tuned, and enhanced, much like the car at the mechanic’s shop. As we draw back the curtains on the “black boxes,” my hope is that this clarity propels us toward more efficient, ethical, and insightful applications that benefit us all.
Feel free to explore the site to obtain insight into my projects, publications, and ongoing research. If you have any comments, thoughts or queries, you can reach me at arjun.k018@gmail.com.
news
Oct 4, 2023 | New preprint released! We applied the Episodic Memory Theory to mechanistically intepret RNNs. We fully describe the behavior of RNNs trained on simple tasks and provide a method to interpret the learned parameters and hidden states. Check it out at https://arxiv.org/abs/2310.02430 |
---|---|
Jul 28, 2023 | Presenting Episodic Memory Theory of Recurrent Neural Networks at TAG-ML workshop in ICML 2023, Hawaii. Check-in for an approach to mechanistically interpret RNNs using ideas from the Memory Modeling literature. |
Jul 21, 2023 | Presenting General Sequential Episodic Memory Model at ICML 2023, Hawaii. Looking forward to meet all the memory researchers there. |
Jul 3, 2023 | Presentation and discussions at the Institute for Machine Learning, Johannes Kepler University on GSEMM and EMT. Thanks to Günter, Daniel, Sebastian and the others for a wonderful summer of research. |
Nov 21, 2021 | Check out our new preprint Energy-based General Sequential Episodic Memory Networks at the Adiabatic Limit for a new memory modeling paradigm where the energy surface is not stationary but have temporal characteristics. |
latest posts
Oct 18, 2023 | Fractals |
---|---|
Sep 14, 2023 | Memory and the Energy Paradigm |